File:Fig1 Beaulieu-JonesJMIRMedInfo2018 6-1.png
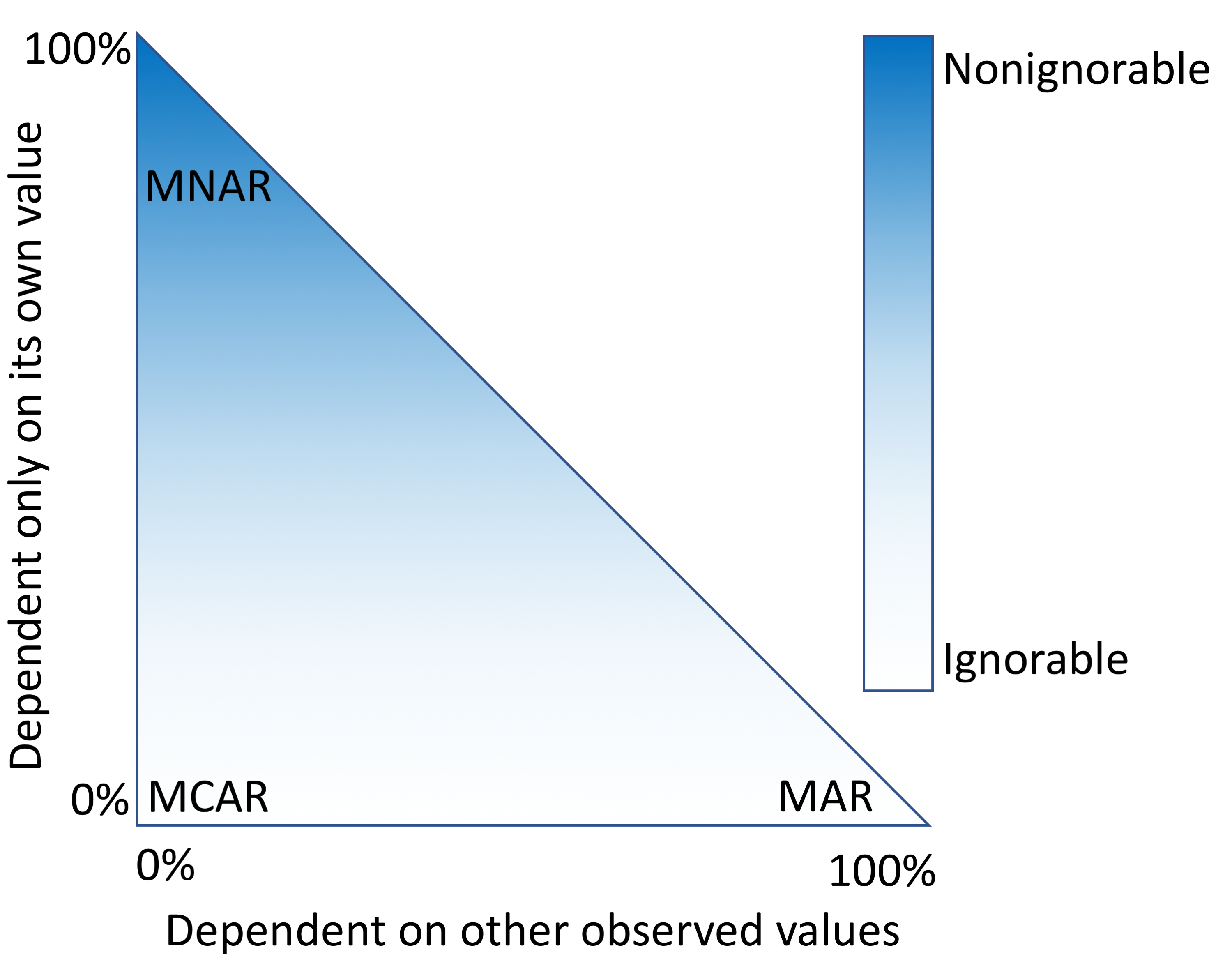
Original file (2,999 × 2,356 pixels, file size: 1.14 MB, MIME type: image/png)
Summary
Description |
Figure 1. Two general paradigms are commonly used to describe missing data. Missing data are considered ignorable if the probability of observing a variable has no relation to the value of the observed variable and are considered nonignorable otherwise. The second paradigm divides missingness into three categories: missing completely at random (MCAR: the probability of observing a variable is not dependent on its value or other observed values), missing at random (MAR: the probability of observing a variable is not dependent on its own value after conditioning on other observed variables), and missing not at random (MNAR: the probability of observing a variable is dependent on its value, even after conditioning on other observed variables). The x-axis indicates the extent to which a given value being observed depends on other values of other observed variables. The y-axis indicates the extent to which a given value being observed depends on its own value. |
---|---|
Source |
Beaulieu-Jones, B.K.; Lavage, D.R.; Snyder, J.W.; Moore, J.H.; Pendergrass, S.A.; Bauer, C.R. (2018). "Characterizing and managing missing structured data in electronic health records: Data analysis". JMIR Medical Informatics 6 (1): e11. doi:10.2196/medinform.8960. |
Date |
2018 |
Author |
Beaulieu-Jones, B.K.; Lavage, D.R.; Snyder, J.W.; Moore, J.H.; Pendergrass, S.A.; Bauer, C.R. |
Permission (Reusing this file) |
|
Other versions |
Licensing
![]() ![]() |
This work is licensed under the Creative Commons Attribution 4.0 License. |
File history
Click on a date/time to view the file as it appeared at that time.
Date/Time | Thumbnail | Dimensions | User | Comment | |
---|---|---|---|---|---|
current | 17:25, 6 March 2018 | ![]() | 2,999 × 2,356 (1.14 MB) | Shawndouglas (talk | contribs) |
You cannot overwrite this file.
File usage
The following 2 pages use this file: